02 - Inference
Estimating latent variables in dynamical systems is a fundamental challenge in data-driven decision-making, particularly when noise characteristics are unknown.
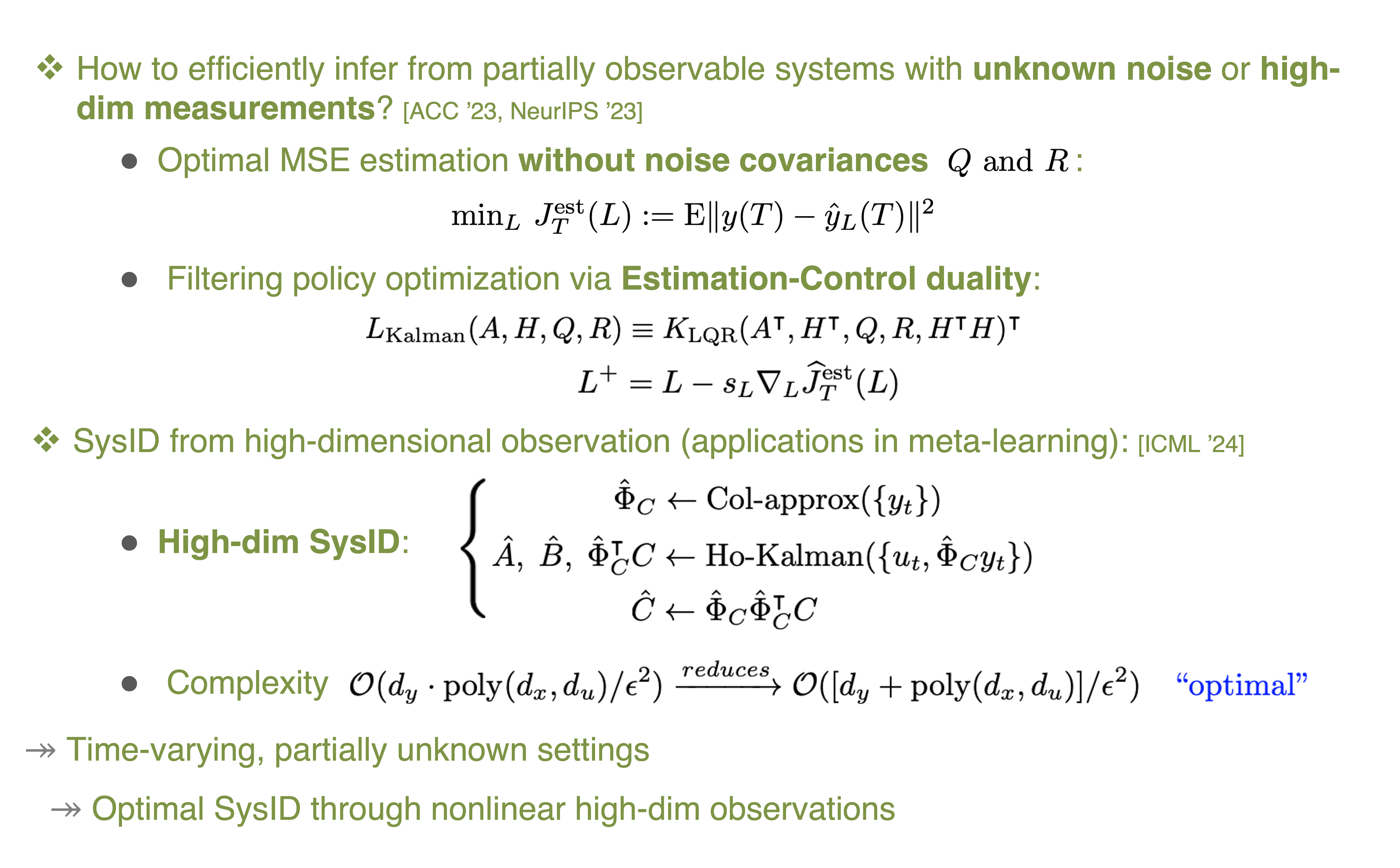
Estimating latent variables is a fundamental challenge in data-driven decision-making, particularly when system characteristics are unknown. The knowledge of noise covariances is often challenging in real-world systems where such statistics are difficult to estimate (Zhang, et. al., IEEE Access 2020). By leveraging the duality between control and estimation, I developed a direct policy optimization framework for optimal estimation in dynamical systems with uncertain dynamics and noisy measurements, even when noise statistics are unknown (Talebi et al., 2023). The contributions include analysis of biased gradient estimators under stability constraints, providing non-asymptotic global convergence guarantees that scale logarithmically with system dimension (Talebi et al., 2023). This work opens the door for data-driven solutions to classical filtering problems, with future directions aimed at nonlinear systems and adaptive filtering in time-varying environments.
Building on Willems' Lemma on a parallel track, I have also extended the characterization of exactly how system-theoretic properties and the excitation of input signals relate to the interoperability of every single input-output trajectory, through a complete analysis of reachable, observable, and initial subspaces (Yu et al., 2021). Our results show that data-driven predictive control using online data is equivalent to model predictive control, even for uncontrollable systems.
Extending these results to large-scale systems where high-dimensional noisy data presents a significant challenge in system identification and inference, such as in neuroscience and finance. In this context, my work (Zhang et al., 2024) makes a significant contribution by addressing the identification of low-dimensional linear time-invariant (LTI) models from high-dimensional noisy observations. We propose a two-stage algorithm that first recovers the high-dimensional observation space and then learns the low-dimensional system dynamics. This achieves an optimal sample complexity and demonstrates that shared structures across systems can further reduce complexity through meta-learning.
Key references:
-
Talebi, S., Taghvaei, A., & Mesbahi, M. (2023). Duality-based stochastic policy optimization for estimation with unknown noise covariances. 2023 American Control Conference (ACC), 622–627.
-
Talebi, S., Taghvaei, A., & Mesbahi, M. (2023). Data-driven Optimal Filtering for Linear Systems with Unknown Noise Covariances. Advances in Neural Information Processing Systems (NeurIPS), 36, 69546–69585.
-
Yu, Y., Talebi, S., & others. (2021). On Controllability and Persistency of Excitation in Data-Driven Control: Ext. of Willems’ Fundamental Lemma. 60th IEEE Conf on Decision and Control , 6485–6490.
-
Zhang, Y., Talebi, S., & Li, N. (2024). Learning Low-dimensional Latent Dynamics from High- dimensional Observations: Non-asymptotics and Lower Bounds. Proc. of the 41st Int Conf on Machine Learning (ICML), 235, 59851–59896.